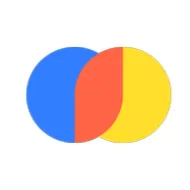
Chroma Integration
Pricing
Pay per usage
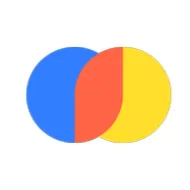
Chroma Integration
This integration transfers data from Apify Actors to a Chroma and is a good starting point for a question-answering, search, or RAG use case.
4.7 (2)
Pricing
Pay per usage
1
Total users
1
Monthly users
1
Last modified
2 months ago
You can access the Chroma Integration programmatically from your own applications by using the Apify API. You can also choose the language preference from below. To use the Apify API, you’ll need an Apify account and your API token, found in Integrations settings in Apify Console.
{ "openapi": "3.0.1", "info": { "version": "0.1", "x-build-id": "ZRWMhPjXSYqdVHl00" }, "servers": [ { "url": "https://api.apify.com/v2" } ], "paths": { "/acts/apify~chroma-integration/run-sync-get-dataset-items": { "post": { "operationId": "run-sync-get-dataset-items-apify-chroma-integration", "x-openai-isConsequential": false, "summary": "Executes an Actor, waits for its completion, and returns Actor's dataset items in response.", "tags": [ "Run Actor" ], "requestBody": { "required": true, "content": { "application/json": { "schema": { "$ref": "#/components/schemas/inputSchema" } } } }, "parameters": [ { "name": "token", "in": "query", "required": true, "schema": { "type": "string" }, "description": "Enter your Apify token here" } ], "responses": { "200": { "description": "OK" } } } }, "/acts/apify~chroma-integration/runs": { "post": { "operationId": "runs-sync-apify-chroma-integration", "x-openai-isConsequential": false, "summary": "Executes an Actor and returns information about the initiated run in response.", "tags": [ "Run Actor" ], "requestBody": { "required": true, "content": { "application/json": { "schema": { "$ref": "#/components/schemas/inputSchema" } } } }, "parameters": [ { "name": "token", "in": "query", "required": true, "schema": { "type": "string" }, "description": "Enter your Apify token here" } ], "responses": { "200": { "description": "OK", "content": { "application/json": { "schema": { "$ref": "#/components/schemas/runsResponseSchema" } } } } } } }, "/acts/apify~chroma-integration/run-sync": { "post": { "operationId": "run-sync-apify-chroma-integration", "x-openai-isConsequential": false, "summary": "Executes an Actor, waits for completion, and returns the OUTPUT from Key-value store in response.", "tags": [ "Run Actor" ], "requestBody": { "required": true, "content": { "application/json": { "schema": { "$ref": "#/components/schemas/inputSchema" } } } }, "parameters": [ { "name": "token", "in": "query", "required": true, "schema": { "type": "string" }, "description": "Enter your Apify token here" } ], "responses": { "200": { "description": "OK" } } } } }, "components": { "schemas": { "inputSchema": { "type": "object", "required": [ "chromaClientHost", "embeddingsProvider", "embeddingsApiKey", "datasetFields" ], "properties": { "chromaCollectionName": { "title": "Chroma collection name", "type": "string", "description": "Name of the chroma collection where the data will be stored", "default": "chroma" }, "chromaClientHost": { "title": "Chroma host", "type": "string", "description": "Host argument for Chroma HTTP Client" }, "chromaClientPort": { "title": "Chroma port", "type": "integer", "description": "Port argument for Chroma HTTP Client", "default": 8000 }, "chromaClientSsl": { "title": "Chroma SSL enabled", "type": "boolean", "description": "Enable/Disable SSL", "default": false }, "chromaServerAuthCredentials": { "title": "Chroma server Auth Static API token credentials", "type": "string", "description": "Chroma server Auth Static API token." }, "chromaClientAuthProvider": { "title": "Chroma client auth provider", "type": "string", "description": "Chroma client auth provider", "default": "chromadb.auth.token_authn.TokenAuthClientProvider" }, "embeddingsProvider": { "title": "Embeddings provider (as defined in the langchain API)", "enum": [ "OpenAI", "Cohere" ], "type": "string", "description": "Choose the embeddings provider to use for generating embeddings", "default": "OpenAI" }, "embeddingsConfig": { "title": "Configuration for embeddings provider", "type": "object", "description": "Configure the parameters for the LangChain embedding class. Key points to consider:\n\n1. Typically, you only need to specify the model name. For example, for OpenAI, set the model name as {\"model\": \"text-embedding-3-small\"}.\n\n2. It's required to ensure that the vector size of your embeddings matches the size of embeddings in the database.\n\n3. Here are examples of embedding models:\n - [OpenAI](https://platform.openai.com/docs/guides/embeddings): `text-embedding-3-small`, `text-embedding-3-large`, etc.\n - [Cohere](https://docs.cohere.com/docs/cohere-embed): `embed-english-v3.0`, `embed-multilingual-light-v3.0`, etc.\n\n4. For more details about other parameters, refer to the [LangChain documentation](https://python.langchain.com/docs/integrations/text_embedding/)." }, "embeddingsApiKey": { "title": "Embeddings API KEY (whenever applicable, depends on provider)", "type": "string", "description": "Value of the API KEY for the embeddings provider (if required).\n\n For example for OpenAI it is OPENAI_API_KEY, for Cohere it is COHERE_API_KEY)" }, "datasetFields": { "title": "Dataset fields to select from the dataset results and store in the database", "type": "array", "description": "This array specifies the dataset fields to be selected and stored in the vector store. Only the fields listed here will be included in the vector store.\n\nFor instance, when using the Website Content Crawler, you might choose to include fields such as `text`, `url`, and `metadata.title` in the vector store.", "default": [ "text" ], "items": { "type": "string" } }, "metadataDatasetFields": { "title": "Dataset fields to select from the dataset and store as metadata in the database", "type": "object", "description": "A list of dataset fields which should be selected from the dataset and stored as metadata in the vector stores.\n\nFor example, when using the Website Content Crawler, you might want to store `url` in metadata. In this case, use `metadataDatasetFields parameter as follows {\"url\": \"url\"}`" }, "metadataObject": { "title": "Custom object to be stored as metadata in the vector store database", "type": "object", "description": "This object allows you to store custom metadata for every item in the vector store.\n\nFor example, if you want to store the `domain` as metadata, use the `metadataObject` like this: {\"domain\": \"apify.com\"}." }, "datasetId": { "title": "Dataset ID", "type": "string", "description": "Dataset ID (when running standalone without integration)" }, "dataUpdatesStrategy": { "title": "Update strategy (add, upsert, deltaUpdates (default))", "enum": [ "add", "upsert", "deltaUpdates" ], "type": "string", "description": "Choose the update strategy for the integration. The update strategy determines how the integration updates the data in the database.\n\nThe available options are:\n\n- **Add data** (`add`):\n - Always adds new records to the database.\n - No checks for existing records or updates are performed.\n - Useful when appending data without concern for duplicates.\n\n- **Upsert data** (`upsert`):\n - Updates existing records if they match a key or identifier.\n - Inserts new records into the database if they don't already exist.\n - Ideal for ensuring the database contains the most up-to-date data, avoiding duplicates.\n\n- **Update changed data based on deltas** (`deltaUpdates`):\n - Performs incremental updates by identifying differences (deltas) between the new dataset and the existing records.\n - Only adds new records and updates those that have changed.\n - Unchanged records are left untouched.\n - Maximizes efficiency by reducing unnecessary updates.\n\nSelect the strategy that best fits your use case.", "default": "deltaUpdates" }, "dataUpdatesPrimaryDatasetFields": { "title": "Dataset fields to uniquely identify dataset items (only relevant when dataUpdatesStrategy is `upsert` or `deltaUpdates`)", "type": "array", "description": "This array contains fields that are used to uniquely identify dataset items, which helps to handle content changes across different runs.\n\nFor instance, in a web content crawling scenario, the `url` field could serve as a unique identifier for each item.", "default": [ "url" ], "items": { "type": "string" } }, "enableDeltaUpdates": { "title": "Enable incremental updates for objects based on deltas (deprecated)", "type": "boolean", "description": "When set to true, this setting enables incremental updates for objects in the database by comparing the changes (deltas) between the crawled dataset items and the existing objects, uniquely identified by the `datasetKeysToItemId` field.\n\n The integration will only add new objects and update those that have changed, reducing unnecessary updates. The `datasetFields`, `metadataDatasetFields`, and `metadataObject` fields are used to determine the changes.", "default": true }, "deleteExpiredObjects": { "title": "Delete expired objects from the database", "type": "boolean", "description": "When set to true, delete objects from the database that have not been crawled for a specified period.", "default": true }, "expiredObjectDeletionPeriodDays": { "title": "Delete expired objects from the database after a specified number of days", "minimum": 0, "type": "integer", "description": "This setting allows the integration to manage the deletion of objects from the database that have not been crawled for a specified period. It is typically used in subsequent runs after the initial crawl.\n\nWhen the value is greater than 0, the integration checks if objects have been seen within the last X days (determined by the expiration period). If the objects are expired, they are deleted from the database. The specific value for `deletedExpiredObjectsDays` depends on your use case and how frequently you crawl data.\n\nFor example, if you crawl data daily, you can set `deletedExpiredObjectsDays` to 7 days. If you crawl data weekly, you can set `deletedExpiredObjectsDays` to 30 days.", "default": 30 }, "performChunking": { "title": "Enable text chunking", "type": "boolean", "description": "When set to true, the text will be divided into smaller chunks based on the settings provided below. Proper chunking helps optimize retrieval and ensures accurate and efficient responses.", "default": true }, "chunkSize": { "title": "Maximum chunk size", "minimum": 1, "type": "integer", "description": "Defines the maximum number of characters in each text chunk. Choosing the right size balances between detailed context and system performance. Optimal sizes ensure high relevancy and minimal response time.", "default": 2000 }, "chunkOverlap": { "title": "Chunk overlap", "minimum": 0, "type": "integer", "description": "Specifies the number of overlapping characters between consecutive text chunks. Adjusting this helps maintain context across chunks, which is crucial for accuracy in retrieval-augmented generation systems.", "default": 0 } } }, "runsResponseSchema": { "type": "object", "properties": { "data": { "type": "object", "properties": { "id": { "type": "string" }, "actId": { "type": "string" }, "userId": { "type": "string" }, "startedAt": { "type": "string", "format": "date-time", "example": "2025-01-08T00:00:00.000Z" }, "finishedAt": { "type": "string", "format": "date-time", "example": "2025-01-08T00:00:00.000Z" }, "status": { "type": "string", "example": "READY" }, "meta": { "type": "object", "properties": { "origin": { "type": "string", "example": "API" }, "userAgent": { "type": "string" } } }, "stats": { "type": "object", "properties": { "inputBodyLen": { "type": "integer", "example": 2000 }, "rebootCount": { "type": "integer", "example": 0 }, "restartCount": { "type": "integer", "example": 0 }, "resurrectCount": { "type": "integer", "example": 0 }, "computeUnits": { "type": "integer", "example": 0 } } }, "options": { "type": "object", "properties": { "build": { "type": "string", "example": "latest" }, "timeoutSecs": { "type": "integer", "example": 300 }, "memoryMbytes": { "type": "integer", "example": 1024 }, "diskMbytes": { "type": "integer", "example": 2048 } } }, "buildId": { "type": "string" }, "defaultKeyValueStoreId": { "type": "string" }, "defaultDatasetId": { "type": "string" }, "defaultRequestQueueId": { "type": "string" }, "buildNumber": { "type": "string", "example": "1.0.0" }, "containerUrl": { "type": "string" }, "usage": { "type": "object", "properties": { "ACTOR_COMPUTE_UNITS": { "type": "integer", "example": 0 }, "DATASET_READS": { "type": "integer", "example": 0 }, "DATASET_WRITES": { "type": "integer", "example": 0 }, "KEY_VALUE_STORE_READS": { "type": "integer", "example": 0 }, "KEY_VALUE_STORE_WRITES": { "type": "integer", "example": 1 }, "KEY_VALUE_STORE_LISTS": { "type": "integer", "example": 0 }, "REQUEST_QUEUE_READS": { "type": "integer", "example": 0 }, "REQUEST_QUEUE_WRITES": { "type": "integer", "example": 0 }, "DATA_TRANSFER_INTERNAL_GBYTES": { "type": "integer", "example": 0 }, "DATA_TRANSFER_EXTERNAL_GBYTES": { "type": "integer", "example": 0 }, "PROXY_RESIDENTIAL_TRANSFER_GBYTES": { "type": "integer", "example": 0 }, "PROXY_SERPS": { "type": "integer", "example": 0 } } }, "usageTotalUsd": { "type": "number", "example": 0.00005 }, "usageUsd": { "type": "object", "properties": { "ACTOR_COMPUTE_UNITS": { "type": "integer", "example": 0 }, "DATASET_READS": { "type": "integer", "example": 0 }, "DATASET_WRITES": { "type": "integer", "example": 0 }, "KEY_VALUE_STORE_READS": { "type": "integer", "example": 0 }, "KEY_VALUE_STORE_WRITES": { "type": "number", "example": 0.00005 }, "KEY_VALUE_STORE_LISTS": { "type": "integer", "example": 0 }, "REQUEST_QUEUE_READS": { "type": "integer", "example": 0 }, "REQUEST_QUEUE_WRITES": { "type": "integer", "example": 0 }, "DATA_TRANSFER_INTERNAL_GBYTES": { "type": "integer", "example": 0 }, "DATA_TRANSFER_EXTERNAL_GBYTES": { "type": "integer", "example": 0 }, "PROXY_RESIDENTIAL_TRANSFER_GBYTES": { "type": "integer", "example": 0 }, "PROXY_SERPS": { "type": "integer", "example": 0 } } } } } } } } }}
Chroma Integration OpenAPI definition
OpenAPI is a standard for designing and describing RESTful APIs, allowing developers to define API structure, endpoints, and data formats in a machine-readable way. It simplifies API development, integration, and documentation.
OpenAPI is effective when used with AI agents and GPTs by standardizing how these systems interact with various APIs, for reliable integrations and efficient communication.
By defining machine-readable API specifications, OpenAPI allows AI models like GPTs to understand and use varied data sources, improving accuracy. This accelerates development, reduces errors, and provides context-aware responses, making OpenAPI a core component for AI applications.
You can download the OpenAPI definitions for Chroma Integration from the options below:
If you’d like to learn more about how OpenAPI powers GPTs, read our blog post.
You can also check out our other API clients: